Are you looking to improve your decision-making skills for your business? Do you want to better understand the dependencies between two events for the same?
If your answer to both of these questions is yes, then you are on the right page, as this article discusses conditional probability.
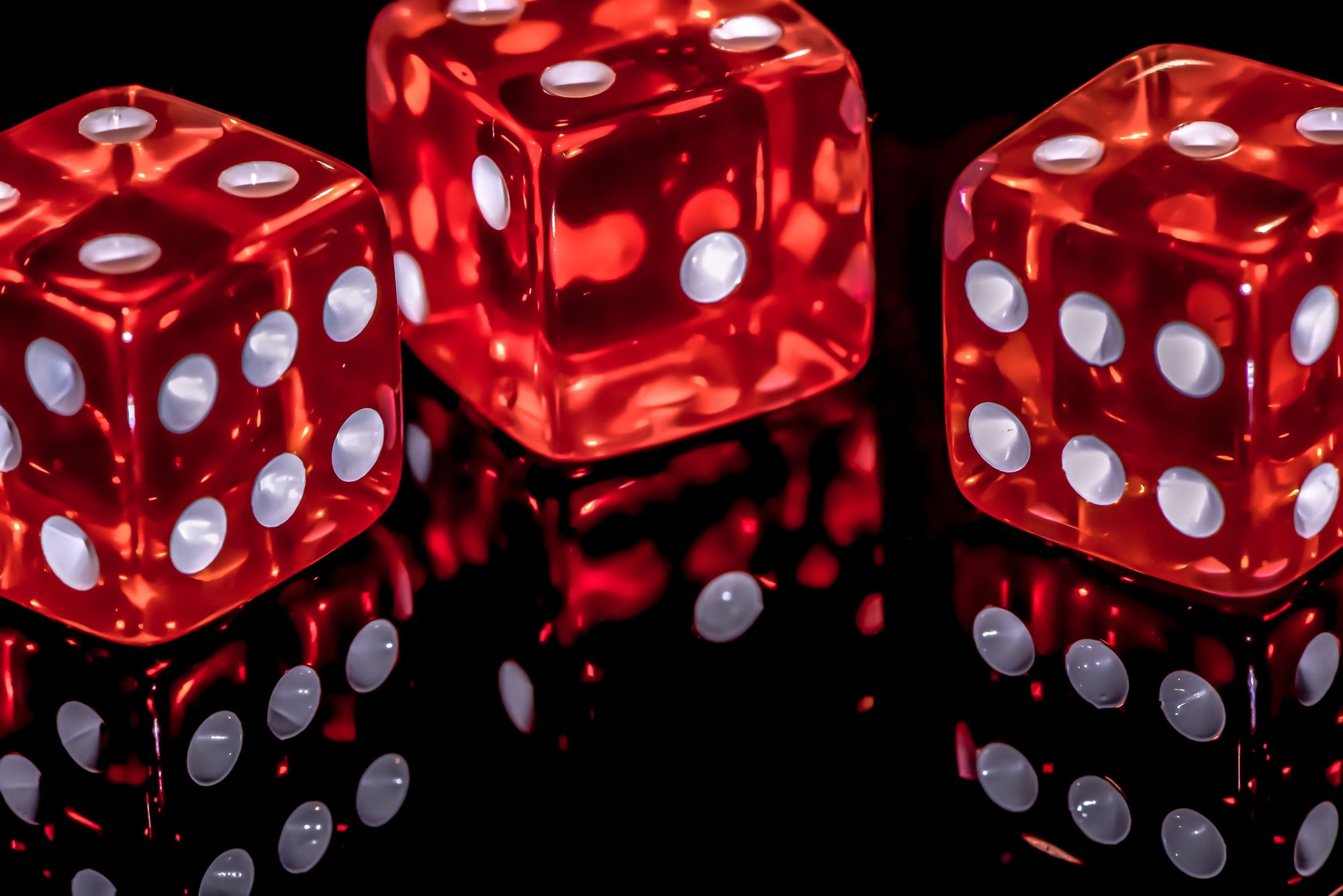
Conditional probability is the probability of an event occurring, given that another event has already occurred. It is calculated by dividing the probability of the two events occurring together by the probability of the first event occurring.
Thus, understanding conditional probability will help your business not only maximize its revenue and net profit ratio but also help you improve your cash flow. In fact, a better understanding of conditional probability will ensure the success of your business.
To help you understand all about conditional probability, this article will cover the following topics:
- What is Conditional Probability?
- The Formula of Conditional Probability
- Differentiate between Conditional Probability vs. Unconditional Probability
- Differentiate between Conditional Probability vs. Joint Probability
- Examples of Conditional Probability
- Bayes’ Theorem and Conditional Probability
- What are the Benefits of Using Conditional Probability in Business?
- What is a Conditional Probability Calculator?
- What is Prior Probability?
- What is Compound Probability?
- FAQs Related to Conditional Probability
- How can Deskera Help in Calculating Conditional Probability?
- Key Takeaways
- Related Articles
What is Conditional Probability?
Conditional probability is defined as the likelihood of an event or outcome occurring based on the occurrence of a previous event or outcome. Thus, conditional probability is calculated by multiplying the probability of the preceding event by the updated probability of the succeeding or conditional event.
This highlights how conditional probability is dependent on a previous event or results occurring. In fact, in conditional probability, such events that are in a relationship with another are looked into.
When one event's occurrence has no impact on the likelihood of the other event occurring, two occurrences are said to be independent. However, two events are considered to be dependent if one event's occurrence or non-occurrence actually influences the likelihood that the other event will occur.
The likelihood of event B does not depend on what happens with event A if the occurrences are independent. Consequently, an event that depends on another has a conditional probability.
Conditional probability is an important concept in statistics and probability theory. It is used in many areas of study, including genetics, finance, engineering, and social sciences, to name just a few.
The Formula of Conditional Probability
Conditional probability is often portrayed as the "probability of A given B," notated as P(A|B).
Considering conditional probability is a way of calculating the probability of one of these events given that we know the other has already occurred. Thus, it is the probability of event A given that event B has occurred. This can be written as:
P(A|B) = P(A and B) / P(B)
Where P(A and B) is the joint probability of events A and B occurring together, and P(B) is the probability of event B occurring.
Let's take an example to illustrate conditional probability. Suppose we have a bag that contains three red balls and two blue balls. We randomly draw one ball from the bag without replacement. What is the probability that the ball is red, given that we know it is not blue?
To solve this problem, we can use conditional probability. Let A be the event that we draw a red ball, and B be the event that we do not draw a blue ball. Then:
P(A|B) = P(A and B) / P(B)
The probability of not drawing a blue ball is the probability of drawing a red ball or the other blue ball, which is 3/5. The joint probability of drawing a red ball and not drawing a blue ball is 3/5 * 2/4 = 3/10 (since there are two blue balls left in the bag after we draw one ball). Therefore, the conditional probability of drawing a red ball given that we do not draw a blue ball is:
P(A|B) = 3/10 / 3/5 = 1/2
So, the probability of drawing a red ball, given that we know it is not blue, is 1/2 or 50%.
Differentiate between Conditional Probability vs. Unconditional Probability
Probability is the measure of the likelihood of an event occurring. Conditional probability and unconditional probability are two types of probability that are often used in statistics and probability theory.
Unconditional probability, also known as marginal probability, refers to the probability of an event occurring without any additional information or conditions. It is calculated as the ratio of the number of favorable outcomes to the total number of possible outcomes.
Conditional probability, on the other hand, is the probability of an event occurring, given that another event has occurred. It is calculated as the ratio of the number of favorable outcomes to the total number of possible outcomes, but only among the events that satisfy the condition.
The notation for conditional probability is P(A|B), where A is the event of interest and B is the condition. For example, P(A|B) would be the probability of event A occurring, given that event B has occurred.
In summary, the unconditional probability is the probability of an event occurring without any additional information, while conditional probability is the probability of an event occurring given that another event has occurred.
Differentiate between Conditional Probability vs. Joint Probability
Conditional probability and joint probability are both concepts in probability theory, but they are different in nature and serve different purposes.
Joint probability refers to the probability of two or more events occurring simultaneously. Thus, it is the probability of the intersection of two or more events.
The probability of the intersection of A and B may be written p(A ∩ B). For example, if you toss two coins at the same time, the joint probability of getting heads on both coins is the probability that both events occur together, which is 1/4 (assuming the coins are fair).
Conditional probability, on the other hand, is the probability of one event occurring, given that another event has already occurred.
For example, if you have two bags of marbles, one containing 3 red and 2 blue marbles and the other containing 2 red and 1 blue marble, and you randomly select a bag and then randomly select a marble from the chosen bag, the probability of selecting a red marble given that you have chosen the first bag is 3/5, and the probability of selecting a red marble given that you have chosen the second bag is 2/3.
Conditional probability is calculated using Bayes' theorem, which relates the conditional probability of an event A given an event B to the joint probability of A and B and the marginal probability of B. It is often used in statistical inference and decision-making.
In summary, joint probability describes the probability of two or more events occurring together, while conditional probability describes the probability of one event occurring given that another event has already occurred.
Examples of Conditional Probability
To better understand conditional probability, let’s see some examples illustrating the same:
Coin Toss Example
Suppose you have two coins in a bag, one is fair and the other is biased towards tails, meaning it has a higher probability of landing on tails. If you pick a coin at random and flip it, the probability of getting heads is different depending on which coin you pick.
If we let A be the event that the coin is biased and B be the event that the result of the flip is heads, then the probability of B given A is less than the probability of B given the complementary event, not A. That is P(B|A) < P(B|not A).
Medical Example
Suppose you are given the results of a medical test that screens for a certain disease. If you test positive, the test has a certain false positive rate, meaning there is a chance that you do not actually have the disease.
If we let A be the event that you have the disease and B be the event that you test positive, then the probability of A given B (the probability that you actually have the disease given that you tested positive) is different from the probability of A without knowing the result of the test. That is, P(A|B) is different from P(A).
Weather Example
Suppose you want to know the probability that it will rain tomorrow, given that it rained today. If we let A be the event that it will rain tomorrow and B be the event that it rained today, then the probability of A given B depends on the weather patterns in your area.
In some areas, if it rained today, the probability of rain tomorrow may be higher than if it did not rain today. In other areas, the opposite may be true. Therefore, the conditional probability of rain tomorrow, given that it rained today, may be different from the unconditional probability of rain tomorrow.
Business Example
One business example of conditional probability is the probability of a customer making a purchase, given that they have added items to their cart.
Suppose an e-commerce company wants to understand how likely a customer is to complete a purchase after adding items to their cart. The company can track the behavior of customers who add items to their cart but do not complete a purchase. Then, they can calculate the conditional probability of purchase, given that items have been added to the cart.
Let A be the event that a customer makes a purchase, and B be the event that the customer has added items to their cart. Then, the conditional probability of a purchase given that items have been added to the cart can be calculated as:
P(A|B) = P(A and B) / P(B)
The probability of A and B is the joint probability of the customer making a purchase and adding items to their cart. The probability of B is the marginal probability of adding items to the cart.
By analyzing the data, the e-commerce company may find that the conditional probability of a purchase, given that items have been added to the cart, is relatively high, which suggests that there is a good chance that customers who add items to their cart will eventually make a purchase.
The company can use this information to optimize its website and marketing strategies, such as offering discounts to customers who have items in their cart but have not yet made a purchase or sending reminder emails to prompt them to complete their purchase. This can ultimately lead to increased sales and net revenue for the company.
Bayes’ Theorem and Conditional Probability
Bayes' theorem is a formula in probability theory that relates the conditional probabilities of two events. It allows us to update our beliefs about the probability of an event based on new information or evidence.
Bayes' theorem is closely related to conditional probability, which is the probability of an event occurring given that another event has already occurred.
Bayes' theorem states that the probability of an event A given an event B is equal to the probability of event B given event A multiplied by the prior probability of event A divided by the prior probability of event B. In symbols:
P(A|B) = P(B|A) * P(A) / P(B)
where P(A|B) is the conditional probability of event A given event B, P(B|A) is the conditional probability of event B given event A, P(A) is the prior probability of event A, and P(B) is the prior probability of event B.
Bayes' theorem can be used in a wide range of applications, such as medical diagnosis, spam filtering, and image recognition.
For example, in medical diagnosis, the prior probability may be the prevalence of a disease in the population, and the conditional probability may be the accuracy of a medical test. By using Bayes' theorem, we can update our beliefs about the probability of a disease given the results of a medical test.
Conditional probability, on the other hand, is the probability of an event occurring, given that another event has already occurred. It is often used in conjunction with Bayes' theorem to compute the posterior probability of an event.
For example, in medical diagnosis, we may use conditional probability to compute the probability of a positive test given that a patient has the disease and the probability of a negative test given that a patient does not have the disease.
In summary, Bayes' theorem and conditional probability are closely related concepts in probability theory that are used to update our beliefs about the probability of an event based on new information or evidence.
Bayes' theorem is a formula that relates the conditional probabilities of two events, while conditional probability is the probability of an event occurring given that another event has already occurred.
What are the Benefits of Using Conditional Probability in Business?
Conditional probability is a powerful tool for decision-making in business and has numerous benefits for businesses looking to improve their decision-making processes. Here are a few of the key benefits of using conditional probability in business:
Improved Risk Assessment
Conditional probability can be used to assess the likelihood of future events based on current or past data. By analyzing data and calculating the probability of a particular event occurring, businesses can make more informed decisions about the risks associated with different business decisions.
For example, businesses can use conditional probability to assess the likelihood of default on a loan or the probability of a product failure.
Based on this assessment, they would be able to accurately set up their safety stock levels, as well as reorder points in inventory. It will also ensure that the needs of the customers are satisfied, which will lead to improved customer retention as well as customer loyalty.
Enhanced Predictive Capabilities
By using conditional probability to analyze past data, businesses can better predict future trends and outcomes.
This can be especially useful in industries where trends change rapidly or where there is a large amount of uncertainty, such as in the financial industry or in the prediction of customer behavior.
With the help of conditional probability, businesses can anticipate the outcomes of various events or actions and plan accordingly.
In fact, with conditional probability, you would be able to better predict the bullwhip effect in supply chains, which will, in turn, lead to more efficient supply chain management.
Additionally, you would also be able to undertake more accurate demand planning, production planning and scheduling, shop floor scheduling, as well as the creation of a master production schedule.
This is also because you would be able to better understand the changes in buyer personas, for example, their preference towards sustainability. This will help you make necessary changes in your suppliers and supply chain such that you are able to encourage returning customers.
Improved Marketing Strategies
Conditional probability can help businesses to better target their marketing efforts by predicting the probability of a customer taking a particular action based on past behavior.
For example, by analyzing past data on customer behavior, businesses can identify patterns and trends that may indicate which marketing campaigns are likely to be most effective for specific customer segments.
Designing your marketing strategies based on these insights will not only help you in achieving your marketing objectives but also improve your marketing KPIs, supported by increased sales velocity and returns on investment.
Better Resource Allocation
Conditional probability can help businesses make better decisions about allocating resources by predicting the probability of different outcomes based on current data.
These insights will thus help in undertaking more effective resource capacity planning, such that all their customers are satisfied, expenses are minimized, and business processes are most productive and profitable.
This can be especially useful for businesses with limited resources or those operating in highly competitive industries. By allocating resources more effectively, businesses can optimize their operations and improve their bottom line.
They can also reduce their operating costs, which in turn will ensure that there is no undue pressure on their working capital. In fact, better resource allocation will lead to improved business metrics and operational metrics.
Enhanced Product Development
Conditional probability can also be used to improve product development by predicting the probability of product success based on past data. By analyzing data on product performance, customer behavior, and market trends, businesses can identify opportunities to improve their product offerings and increase their market share.
In fact, it will also help during the business efforts of continuous innovation through the help of AR, IoT, etc., to improve the product and make it better as well as more cost-effective.
What is a Conditional Probability Calculator?
A conditional probability calculator is a tool or an online application that can be used to calculate the conditional probability of an event occurring, given that another event has already occurred.
These calculators are often used in statistics, probability theory, and machine learning to make predictions or decisions based on existing data or evidence.
A conditional probability calculator usually requires the user to input the prior probabilities of the two events and the conditional probability of one event given the other. It then uses Bayes' theorem to compute the posterior probability of the event of interest. The output of the calculator is that the other event has already occurred.
For example, suppose we want to calculate the probability of a person having a disease given that they have tested positive for the disease. Let A be the event that the person has the disease and B be the event that the person has tested positive.
The conditional probability of a positive test given that the person has the disease is the sensitivity of the test, while the conditional probability of having the disease given a positive test is the positive predictive value of the test.
Using a conditional probability calculator, we can input the sensitivity, the prior probability of having the disease, and the prior probability of a positive test, and compute the positive predictive value of the test.
Conditional probability calculators can be useful in various fields, such as finance, engineering, and healthcare, where making predictions or decisions based on existing data is important. They can help researchers and practitioners to analyze and interpret data and to make informed decisions based on the available evidence.
What is Prior Probability?
In probability theory and statistics, prior probability refers to the probability of an event occurring before any new information or data is taken into account. It is a subjective probability that is based on the available knowledge or beliefs about the event prior to observing any evidence or data. The term "prior" implies that the probability is assigned before any new information is taken into account.
Prior probability can be based on various sources of information, such as historical data, expert opinions, or intuition. For example, if we want to estimate the probability of a coin landing heads up when tossed, and we have no prior knowledge of the coin, we might assume that the probability is 0.5 based on the assumption that the coin is fair.
Prior probability is often updated by new information or data using Bayes' theorem, which allows us to calculate the posterior probability of an event given the observed data. The posterior probability is a revised probability that incorporates the prior probability and the new evidence or data.
Prior probability is important in many areas of research and decision-making, such as Bayesian statistics, machine learning, and risk analysis. It allows us to make informed decisions based on the available information and to update our beliefs as new information becomes available.
What is Compound Probability?
Compound probability refers to the probability of two or more events occurring together or in sequence. It is the probability of a joint event or the probability of multiple events occurring simultaneously.
There are two types of compound probability: dependent and independent. In dependent probability, the occurrence of one event affects the probability of the other event. In independent probability, the occurrence of one event does not affect the probability of the other event.
The calculation of compound probability depends on whether the events are dependent or independent. If the events are independent, we can use the multiplication rule, which states that the probability of two independent events occurring together is the product of their individual probabilities.
For example, if the probability of rolling a 4 on a fair six-sided die is 1/6, and the probability of rolling a 5 is also 1/6, then the probability of rolling a 4 and a 5 on two consecutive rolls is (1/6) * (1/6) = 1/36.
If the events are dependent, we need to use conditional probability to calculate the compound probability. The conditional probability of an event is the probability of the event given that another event has already occurred.
For example, if the probability of a student passing a math test is 0.7, and the probability of passing an English test is 0.6, then the probability of passing both tests is the conditional probability of passing English given that the student has already passed math, multiplied by the probability of passing math. If we assume that passing math is a prerequisite for passing English, then the probability of passing both tests is 0.7 * 0.6 = 0.42.
Compound probability is an important concept in probability theory and is used in many areas of research and decision-making, such as finance, engineering, and healthcare. It helps us to calculate the probability of multiple events occurring together or in sequence, which can help us to make informed decisions based on the available information.
FAQs Related to Conditional Probability
- What is conditional probability?
Conditional probability is the probability of an event occurring, given that another event has already occurred. It is the likelihood of an event occurring based on some additional information that is known.
- How is a conditional probability calculated?
Conditional probability is calculated by dividing the probability of the intersection of two events by the probability of the event that has already occurred. The formula for conditional probability is P(A|B) = P(A and B) / P(B).
- What is Bayes' theorem?
Bayes' theorem is a mathematical formula used to calculate conditional probabilities. It provides a way to update the probability of an event based on new information or evidence.
- What is the difference between dependent and independent events?
In dependent events, the occurrence of one event affects the probability of the other event. In independent events, the occurrence of one event does not affect the probability of the other event.
- What is the multiplication rule?
The multiplication rule is a rule used to calculate the probability of two independent events occurring together. It states that the probability of two independent events occurring together is the product of their individual probabilities.
- What is the addition rule?
The addition rule is a rule used to calculate the probability of either of two mutually exclusive events occurring. It states that the probability of either of two mutually exclusive events occurring is the sum of their individual probabilities.
- What is a tree diagram?
A tree diagram is a graphical representation used to calculate the probability of multiple events occurring in sequence. It is a useful tool for visualizing the different possible outcomes of a series of events.
- What is the law of total probability?
The law of total probability is a mathematical formula used to calculate the probability of an event occurring. It states that the probability of an event is the sum of the probabilities of the event occurring under different conditions.
- What is the difference between prior probability and posterior probability?
Prior probability is the probability of an event occurring before any new information or data is taken into account. The posterior probability is the revised probability that incorporates the prior probability and the new evidence or data.
- How is conditional probability used in real-life situations?
Conditional probability is used in a wide range of real-life situations, such as weather forecasting, medical diagnosis, and financial analysis. It helps to make informed decisions based on the available information and to update our beliefs as new information becomes available.
How can Deskera Help in Calculating Conditional Probability?
Deskera is an all-in-one business management software that offers a range of tools and features to help businesses with accounting, inventory management, customer relationship management, human resources management, and more.
While Deskera does not have a specific built-in feature for calculating conditional probabilities, it can be used to input and analyze data that can be used in conditional probability calculations.
For example, Deskera Books can be used to track sales, expenses, and other financial data, which can be used to calculate the probability of various events occurring, such as the probability of a customer making a purchase or the probability of a particular product being in stock.
Deskera CRM can also be used to track inventory levels and sales data, which can be used to calculate the probability of selling a certain number of products within a given time frame.
Deskera People can be used to track the efficiency as well as the time taken by employees to complete certain tasks. It will also help in predicting holidays as well as overtime so that businesses can plan their processes accordingly.
Additionally, Deskera's reporting and analytics tools can be used to analyze data and generate insights that can inform conditional probability calculations.
By inputting data and analyzing it with Deskera's tools, businesses can gain a better understanding of the factors that influence the probability of certain events occurring, which can help them to make more informed decisions.
Key Takeaways
Conditional probability is a statistical concept that measures the probability of event A, given that another event B has occurred. It is denoted by P(A|B), and it represents the probability of event A, given that event B has occurred.
The formula of conditional probability is:
P(A|B) = P(A and B) / P(B)
Where P(A and B) is the joint probability of events A and B occurring together, and P(B) is the probability of event B occurring.
The benefits of using conditional probability in businesses are:
- Improved Risk Assessment
- Enhanced Predictive Capabilities
- Improved Marketing Strategies
- Better Resource Allocation
- Enhanced Product Development
While Deskera does not provide a specific conditional probability calculator, its various features and tools can be used to input and analyze data that can be used in conditional probability calculations.
This can help businesses to gain insights into the probability of various events occurring and to make data-driven decisions based on the available information.
Related Articles
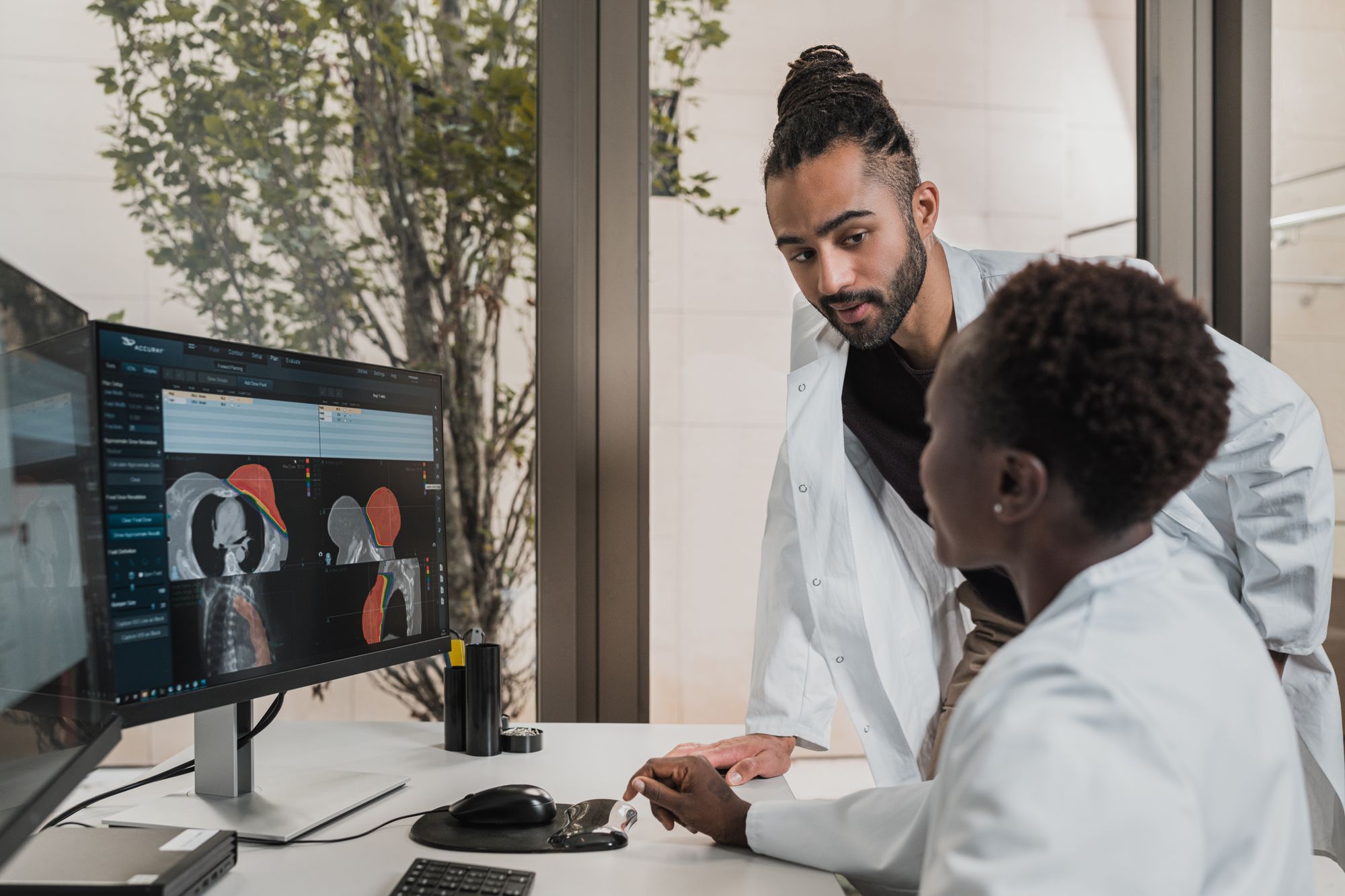
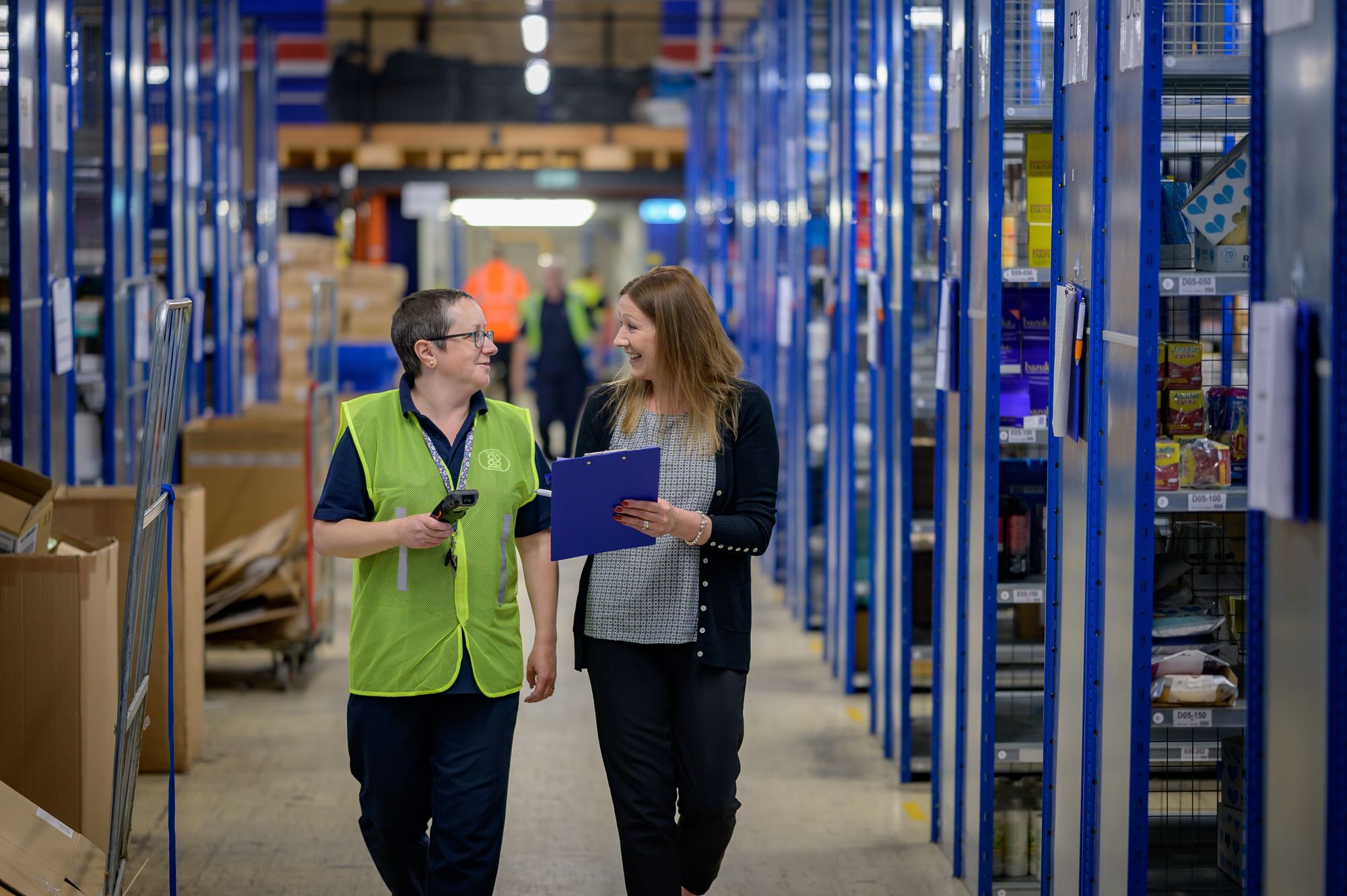
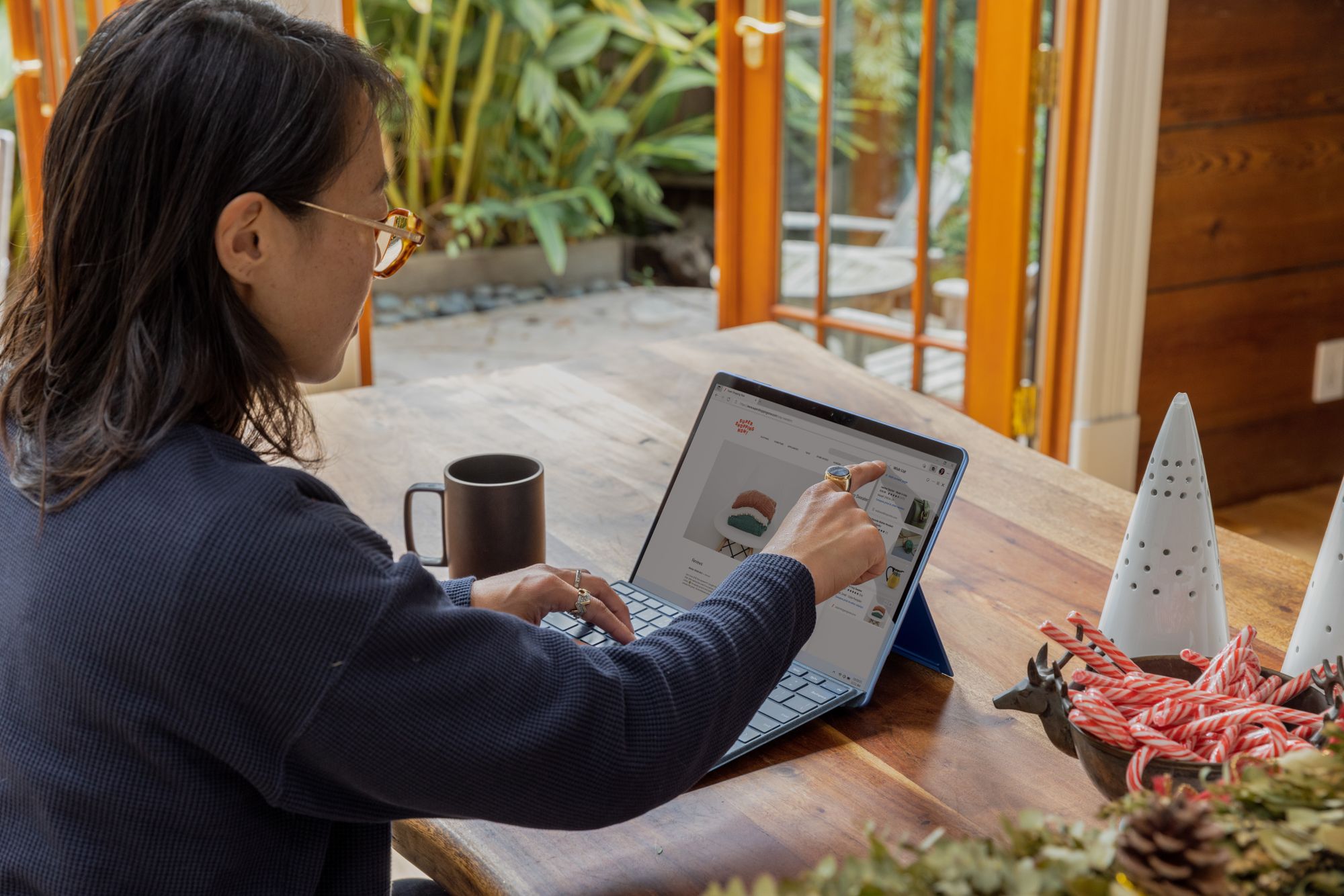
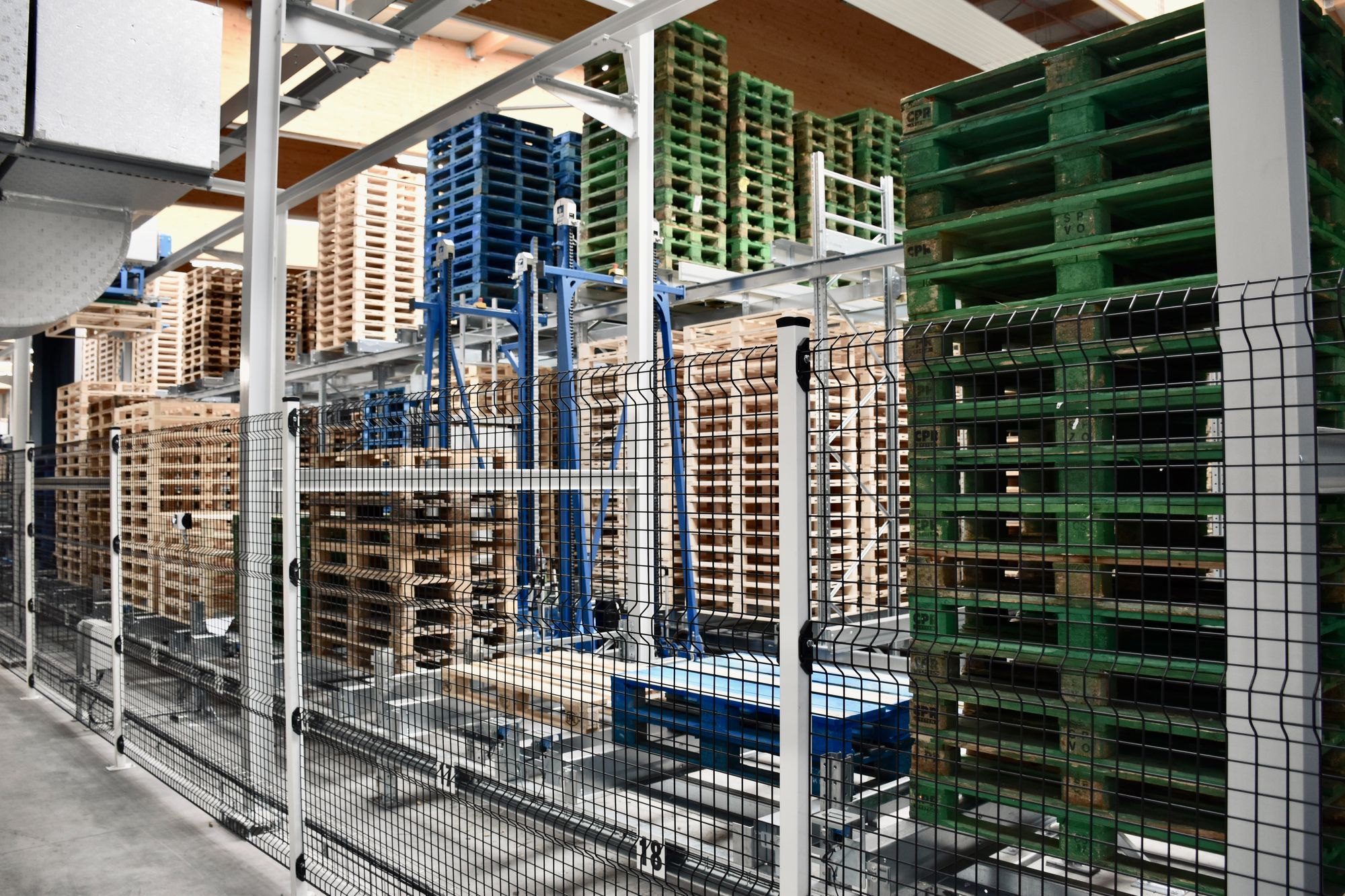
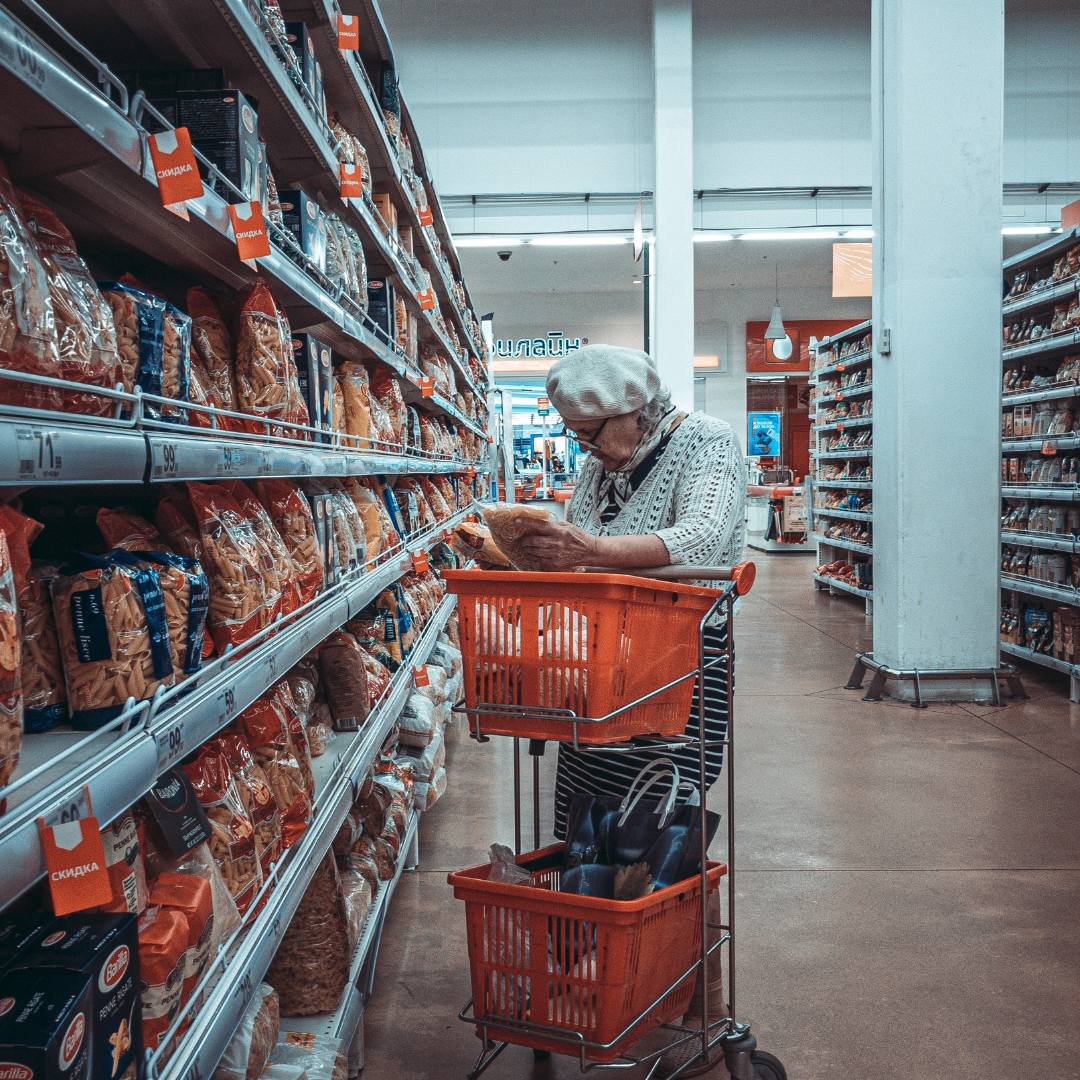
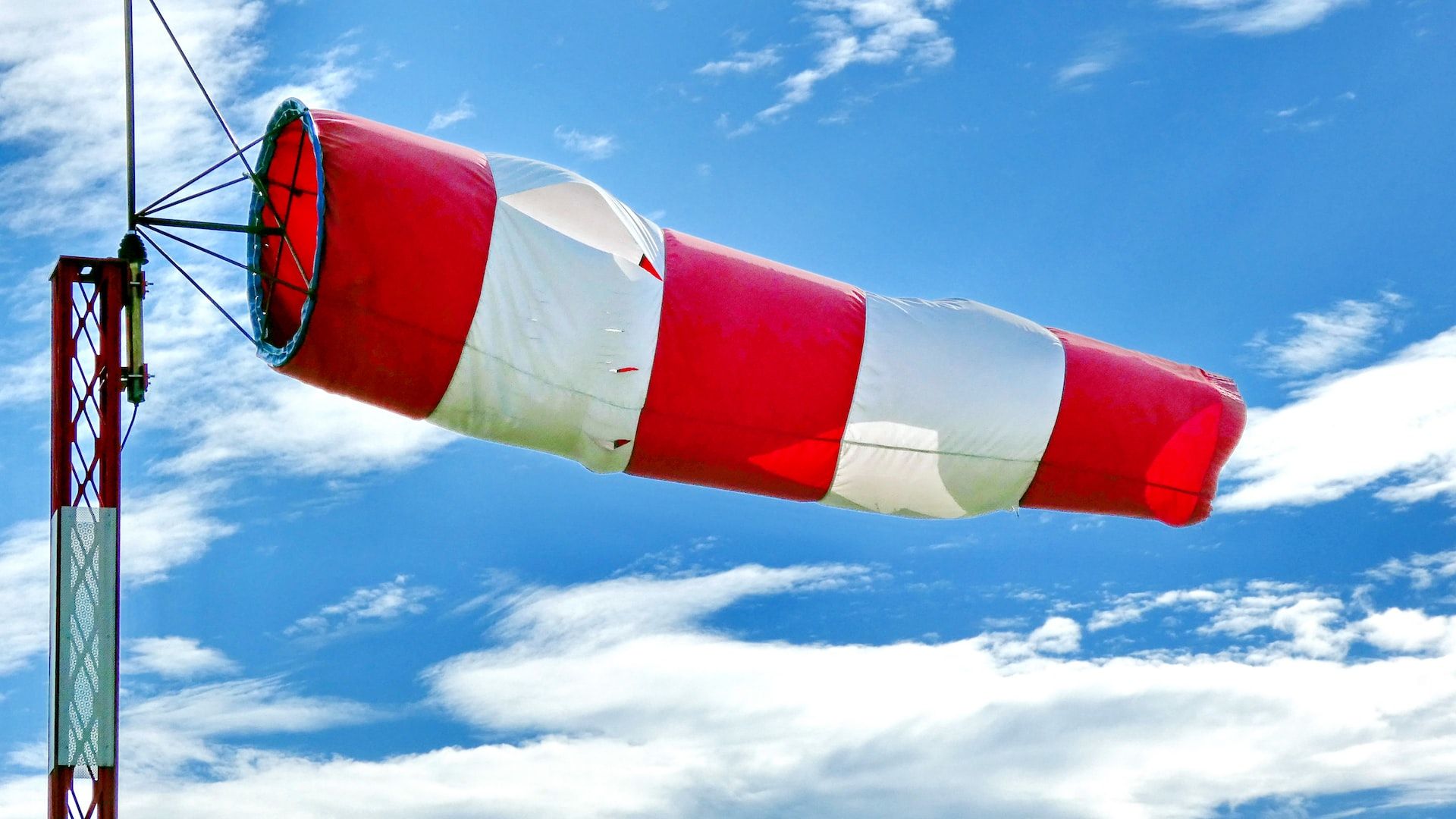