Demand forecasting plays a critical role in the success of manufacturing operations. By accurately predicting customer demand, manufacturers can optimize production plans, streamline inventory management, and enhance overall operational efficiency.
However, the challenges inherent in demand forecasting pose significant hurdles that must be overcome to achieve accurate and actionable forecasts.
In order to be successful in manufacturing, it is essential to be able to forecast future demand. However, this is a difficult task given the myriad of variables that can affect demand.
In this article, we delve into the complexities of demand forecasting in manufacturing and explore the best practices and strategies that can help manufacturers navigate these challenges.
Let’s take a look at the table of content below:
- Meaning of Demand Forecasting in Manufacturing
- Importance of Demand Forecasting in Manufacturing
- Challenges Faced in Demand Forecasting
- Best Practices for Demand Forecasting in Manufacturing
- Strategies for Effective Demand Forecasting
- Frequently Asked Questions (FAQs) Associated with Demand Forecasting in Manufacturing
- Wrapping Up
- How Deskera Can Assist You?
Let's get started!
Meaning of Demand Forecasting in Manufacturing
Demand forecasting in manufacturing refers to the process of estimating future customer demand for a manufacturer's products or services.
Furthermore, it involves analyzing historical data, market trends, and other relevant factors to predict the quantity of products that will be needed within a specific time frame.
Moreover, demand forecasting aims to assist manufacturers in making informed decisions. This includes production planning, inventory management, resource allocation, and overall business strategy.
By accurately forecasting demand, manufacturers can optimize their operations, minimize costs, meet customer expectations, and maintain a competitive edge in the market.
Importance of Demand Forecasting in Manufacturing
Demand forecasting plays a crucial role in manufacturing for several reasons:
Production Planning: Accurate demand forecasting allows manufacturers to plan their production schedules effectively. It helps determine the quantity and timing of production runs, ensuring that sufficient inventory is available to meet customer demands while avoiding excess inventory or stockouts.
Inventory Management: Demand forecasting helps manufacturers optimize their inventory levels. By predicting future demand, they can maintain the right amount of stock on hand, minimizing carrying costs and reducing the risk of stock obsolescence or shortage.
Supply Chain Efficiency: Demand forecasting enables manufacturers to streamline their supply chain operations. With accurate forecasts, they can work closely with suppliers, ensuring timely delivery of raw materials and components, reducing lead times, and improving overall supply chain efficiency.
Cost Reduction: Effective demand forecasting helps manufacturers reduce costs in various ways. By aligning production with demand, they can minimize waste, avoid overproduction, and optimize resource utilization. Additionally, it enables effective negotiation with suppliers and helps manufacturers take advantage of bulk purchasing discounts.
Customer Satisfaction: Meeting customer demands is critical for maintaining customer satisfaction and loyalty. Demand forecasting helps manufacturers ensure product availability, timely delivery, and accurate order fulfillment, leading to improved customer satisfaction and retention.
Financial Planning: Accurate demand forecasts provide manufacturers with valuable insights for financial planning and budgeting. It helps them estimate revenue, allocate resources effectively, plan investments, and manage cash flow more efficiently.
Strategic Decision-Making: Demand forecasting serves as a foundation for strategic decision-making in manufacturing. It enables manufacturers to identify market trends, anticipate changes in customer preferences, and make informed decisions about product development, expansion, market entry, or discontinuation.
In summary, demand forecasting in manufacturing is vital for efficient production planning, optimized inventory management, streamlined supply chain operations, cost reduction, customer satisfaction, financial planning, and strategic decision-making. It empowers manufacturers to respond proactively to market dynamics, enhance competitiveness, and achieve long-term business success.
Challenges Faced in Demand Forecasting
Demand forecasting in manufacturing faces several challenges, which can impact the accuracy and reliability of forecasts. Here are some of the critical challenges in detail:
Volatility and Uncertainty in Demand: Fluctuations in customer demand can be unpredictable and volatile, making it challenging to forecast accurately. External factors such as economic conditions, market trends, competitor actions, and consumer behavior can significantly impact demand patterns. Rapid changes in customer preferences, emerging technologies, or unexpected events (e.g., natural disasters, political disruptions) can further increase demand volatility and uncertainty.
Seasonality and Market Trends: Many industries experience seasonal fluctuations in demand due to factors like holidays, weather conditions, or annual trends.
Furthermore, incorporating seasonality into demand forecasting requires identifying historical patterns, adjusting forecasts accordingly, and accurately predicting variations in demand during different seasons or specific periods.
Additionally, capturing and adapting to market trends, such as evolving consumer preferences or emerging product innovations, can be challenging but crucial for accurate demand forecasting.
Lack of Accurate Historical Data: Demand forecasting heavily relies on historical data to identify patterns, trends, and seasonality. However, obtaining accurate and relevant historical data can be challenging, especially for new products, markets, or rapidly changing industries.
Moreover, insufficient historical data or data that is inconsistent, incomplete, or unreliable can hinder the accuracy of demand forecasts.
External Factors Affecting Demand: Several external factors can influence demand but are beyond the manufacturer's control. Examples include regulatory changes, geopolitical events, natural disasters, economic recessions, or shifts in global trade policies.
Moreover, these factors can significantly impact customer demand, supply chain dynamics, and market conditions, making it challenging to predict and incorporate their effects into demand forecasts.
Demand Fragmentation and Variability: In today's diverse and competitive market, demand fragmentation has become more prominent. Customers have varying preferences, and product customization or personalization has increased.
Furthermore, this fragmentation leads to a wider range of products, SKUs, and demand patterns, making it more challenging to forecast accurately. Additionally, demand variability within product categories or customer segments can add complexity to demand forecasting.
Limited Visibility across the Supply Chain: Demand forecasting is affected by limited visibility and information sharing across the supply chain. Lack of real-time data on inventory levels, production capacities, supplier performance, or customer behavior can result in incomplete or outdated information, leading to suboptimal forecasts. Collaboration and data sharing challenges between departments, suppliers, distributors, and retailers can impact the accuracy of demand forecasts.
Forecasting Errors and Bias: Even with the best forecasting techniques and data, forecasting errors and biases can occur. These errors can stem from inaccuracies in historical data, flawed assumptions, faulty models, or human judgment biases.
Overly optimistic or pessimistic forecasts, inherent biases in forecasting techniques, or inadequate adjustments for outliers or anomalies can result in unreliable demand forecasts.
Addressing these challenges requires employing best practices, leveraging advanced analytical tools, enhancing data collection and sharing processes, incorporating external factors, and adopting agile forecasting strategies. It also requires continuous monitoring, evaluation, and adaptation to improve the accuracy and effectiveness of demand forecasting in manufacturing.
Best Practices for Demand Forecasting in Manufacturing
To overcome the challenges of demand forecasting in manufacturing, implementing best practices is crucial. These practices help improve the accuracy, reliability, and effectiveness of demand forecasting processes.
By following these best practices, manufacturers can make more informed decisions about production planning, inventory management, and overall business strategies. Some key best practices include:
A. Data Collection and Analysis
Data collection and analysis are fundamental steps in demand forecasting in manufacturing. Here are some best practices for data collection and analysis:
Gather Relevant Data Sources: Identify and gather relevant data sources that provide insights into customer demand. This includes historical sales data, customer orders, market research, website analytics, social media data, and industry reports. Incorporate internal and external data sources to capture a comprehensive view of demand drivers.
Clean and Organize Data: Cleanse and preprocess the collected data to remove duplicates, errors, and inconsistencies. Standardize data formats and ensure data integrity. Organize the data in a structured manner to facilitate analysis and forecasting processes.
Utilize Advanced Analytical Tools: Employ advanced analytical techniques and tools to analyze the collected data. Statistical methods, such as time series analysis, regression analysis, and data visualization, can provide valuable insights into demand patterns, trends, seasonality, and correlations with other variables.
Apply Forecasting Models: Utilize appropriate forecasting models based on the characteristics of the data and the specific needs of the manufacturing industry. Common forecasting models include moving averages, exponential smoothing, regression models, and machine learning algorithms. Choose models that align with the nature of the data and have a proven track record of accuracy.
Validate and Refine Models: Validate the forecasting models by comparing their predictions against actual demand data. Assess the accuracy and reliability of the models and refine them if necessary. Furthermore, incorporate feedback from stakeholders and subject matter experts to improve the forecasting models and fine-tune the forecasted results.
Automate Data Collection and Analysis: Leverage automation tools and technologies to streamline data collection and analysis processes. Implement data integration systems, data management platforms, and forecasting software to automate data collection, preprocessing, analysis, and reporting. Automation reduces manual effort, minimizes errors, and enhances efficiency in demand forecasting.
By following these best practices for data collection and analysis, manufacturers can ensure the availability of accurate and reliable data for demand forecasting. This forms the foundation for making informed decisions, optimizing production plans, and effectively managing inventory to meet customer demands in the manufacturing industry.
B. Collaboration between Departments
Collaboration between departments is crucial for effective demand forecasting in manufacturing. By involving cross-functional teams, manufacturers can leverage diverse perspectives, expertise, and data from different areas of the business. Here are some best practices for fostering collaboration between departments:
Engage Sales and Marketing Teams: Sales and marketing teams are at the forefront of understanding customer behavior, market trends, and new product launches. Collaborate with these teams to gather valuable insights on customer preferences, emerging market demands, promotional activities, and customer feedback. Regular communication and joint meetings help align sales and marketing strategies with demand forecasting efforts.
Involve Production and Supply Chain Teams: Collaborate closely with production and supply chain teams to understand production capabilities, capacity constraints, lead times, and inventory levels. Incorporate their expertise in translating demand forecasts into actionable production plans, resource allocation, and inventory management strategies. Regular coordination meetings ensure alignment between demand forecasts and production capabilities.
Incorporate Insights from Finance and Accounting: Finance and accounting departments provide critical financial data and insights that can influence demand forecasting. Collaborate with these departments to incorporate financial metrics, cost analysis, pricing strategies, and budgeting constraints into demand forecasts. This helps align financial goals with demand forecasting outcomes and facilitates informed decision-making.
Foster Communication and Knowledge Sharing: Establish regular communication channels and platforms for collaboration between departments. This can include team meetings, cross-functional workshops, shared databases, or collaboration software. Encourage open dialogue, information sharing, and the exchange of insights and challenges related to demand forecasting. This promotes a collaborative culture and ensures that relevant information flows seamlessly across departments.
Jointly Review and Refine Forecasting Results: Conduct regular meetings or review sessions with stakeholders from different departments to evaluate demand forecasting results. Compare forecasted demand with actual sales data, discuss forecast accuracy, identify gaps or discrepancies, and seek input from various perspectives. Incorporate feedback to refine forecasting models, adjust assumptions, and enhance forecasting methodologies.
Align Incentives and Goals: Aligning incentives and goals across departments can foster collaboration and collective ownership of demand forecasting outcomes. Create a shared understanding of how accurate demand forecasting contributes to overall business success.
Furthermore, consider incorporating demand forecasting accuracy metrics into performance evaluations or reward systems to incentivize collaboration and accountability.
By fostering collaboration between departments, manufacturers can leverage the collective knowledge and expertise of their teams. This leads to more accurate demand forecasts, improved production planning, optimized inventory management, and better decision-making throughout the manufacturing process.
C. Incorporating External Factors
Incorporating external factors into demand forecasting is crucial for capturing the influence of market dynamics, economic conditions, and competitive factors. Here are some best practices for incorporating external factors in detail:
Monitor Market Trends and Competitors: Stay updated on market trends, industry reports, and competitor activities. Monitor changes in consumer preferences, emerging technologies, and new product introductions. Analyze competitor pricing strategies, marketing campaigns, and promotional activities. This information provides valuable insights for adjusting demand forecasts to reflect changing market dynamics.
Consider Economic and Geopolitical Factors: Economic conditions and geopolitical events can significantly impact demand. Monitor economic indicators such as GDP growth, inflation rates, interest rates, and consumer confidence levels. Assess the potential impact of factors like trade policies, government regulations, and geopolitical tensions on customer demand. Adjust demand forecasts accordingly to account for these external influences.
Leverage Technology and Predictive Analytics: Leverage advanced technologies and predictive analytics tools to analyze and incorporate external data sources into demand forecasts.
Utilize machine learning algorithms, data mining techniques, and sentiment analysis to extract insights from social media, news feeds, and online discussions. This helps identify emerging trends, sentiment shifts, and customer preferences that may impact demand.
Engage External Partners and Suppliers: Collaborate with external partners, suppliers, and distributors to gather insights into market demand. Share aggregated data, collaborate on demand planning, and engage in collaborative forecasting efforts.
Furthermore, establish communication channels to exchange information on customer demands, production capacities, and supply chain dynamics. This collaborative approach enhances demand forecasting accuracy by incorporating a broader perspective.
Leverage Market Research and Consumer Insights: Conduct market research studies, surveys, and focus groups to gain deeper insights into customer preferences, purchasing behavior, and expectations. Incorporate customer feedback, reviews, and sentiment analysis to understand evolving consumer trends. These insights help refine demand forecasts by capturing customer-driven factors that may not be evident from internal data alone.
Stay Agile and Adaptive: Recognize that external factors can change rapidly, requiring agility in demand forecasting. Regularly reassess the impact of external factors and update demand forecasts accordingly. Continuously monitor market trends, economic indicators, and competitor activities to stay responsive to evolving market conditions.
Collaborate with Data Providers and Analytics Experts: Partner with data providers or analytics experts specializing in collecting and analyzing external data. These providers can offer access to industry-specific data, market intelligence, and predictive models tailored to manufacturing and demand forecasting needs. Collaborate with them to incorporate their insights and expertise into the demand forecasting process.
By incorporating external factors into demand forecasting, manufacturers can improve the accuracy and relevance of their forecasts. This enables them to make more informed decisions, proactively respond to market changes, and optimize their operations to meet customer demands effectively.
D. Continuous Improvement and Evaluation
Continuous improvement and evaluation are essential for enhancing the accuracy and effectiveness of demand forecasting in manufacturing. Here are some best practices for continuous improvement and evaluation:
Track Forecast Accuracy: Measure the accuracy of demand forecasts by comparing them with actual sales data. Calculate metrics such as mean absolute percentage error (MAPE), forecast bias, or root mean square error (RMSE) to assess forecast performance. Regularly track these metrics to identify areas of improvement and monitor the effectiveness of forecasting techniques.
Analyze Forecast Errors: Analyze the causes of forecast errors and identify patterns or recurring issues. Examine factors such as demand volatility, seasonality, outliers, or unforeseen events that may contribute to forecast inaccuracies. Understanding the sources of errors helps refine forecasting models, adjust assumptions, and incorporate corrective measures.
Incorporate Stakeholder Feedback: Seek feedback from stakeholders involved in the demand forecasting process, including sales teams, marketing teams, production, and supply chain departments. Gather their insights, observations, and challenges related to demand forecasting. Incorporate their feedback into the forecasting process to enhance accuracy and relevance.
Conduct Post-Mortem Reviews: Conduct post-mortem reviews after significant events, product launches, or promotional campaigns. Evaluate the accuracy of demand forecasts for these specific scenarios and identify areas for improvement. Assess the impact of external factors, market trends, or unexpected events on forecast performance and incorporate those insights into future forecasts.
Implement Forecasting System Enhancements: Leverage technology to enhance demand forecasting capabilities. Implement forecasting software or systems that provide advanced analytical capabilities, machine learning algorithms, and automation features. Regularly evaluate and update these systems to ensure they align with evolving business needs and incorporate the latest forecasting methodologies.
Foster a Culture of Learning and Adaptation: Encourage a culture of continuous learning, experimentation, and adaptation within the demand forecasting team. Emphasize the importance of feedback, knowledge sharing, and collaboration. Encourage team members to stay updated on industry trends, attend training programs, or participate in forecasting conferences to enhance their skills and stay informed about emerging forecasting techniques.
Benchmark Against Industry Standards: Benchmark demand forecasting performance against industry standards or best practices. Compare forecast accuracy metrics with industry peers or similar companies to identify areas for improvement. Analyze successful forecasting practices in the industry and explore how they can be applied to enhance your own demand forecasting processes.
By consistently evaluating and improving demand forecasting practices, manufacturers can enhance forecast accuracy, optimize resource allocation, minimize inventory costs, and meet customer demands more effectively. Continuous improvement and evaluation ensure that demand forecasting processes remain dynamic and adaptive to changing market conditions.
Strategies for Effective Demand Forecasting
Effective demand forecasting is crucial for manufacturers to optimize production, manage inventory, and meet customer demands efficiently. To achieve accurate and reliable forecasts, implementing effective strategies is essential.
These strategies encompass various aspects of the demand forecasting process, from data analysis to collaboration and continuous improvement. Here is a brief introduction to some key strategies for effective demand forecasting:
A. Multiple Forecasting Methods
There are several forecasting methods available for demand forecasting in manufacturing. Each method has its own strengths, limitations, and applicability depending on the nature of the data and the specific requirements of the manufacturing industry. Here are some commonly used forecasting methods in detail:
Time Series Analysis:
Moving Averages: Calculates the average of past demand values over a specified period. Simple Moving Average (SMA) assigns equal weights to all data points, while Weighted Moving Average (WMA) assigns different weights based on their importance.
Exponential Smoothing: Assigns exponentially decreasing weights to past demand values, with more recent values having a higher weight. Methods like Single Exponential Smoothing (SES), Double Exponential Smoothing (DES), and Triple Exponential Smoothing (TES) consider different levels of trend and seasonality.
Regression Analysis:
Simple Linear Regression: Establishes a relationship between the dependent variable (demand) and one independent variable (such as price, marketing expenditure, or time). It assumes a linear relationship and predicts demand based on the identified coefficients.
Multiple Regression: Considers multiple independent variables to predict demand, accounting for various factors that may influence demand, such as price, promotion, and external economic indicators.
Seasonal Forecasting:
Seasonal Decomposition: Identifies seasonal patterns by decomposing the demand data into trend, seasonality, and residual components. It helps capture and forecast seasonal fluctuations accurately.
Seasonal Exponential Smoothing: Extends exponential smoothing by incorporating seasonality factors. Models like Holt-Winters' method capture both trend and seasonality components in the data.
Box-Jenkins ARIMA:
Autoregressive Integrated Moving Average (ARIMA): ARIMA models consider the autoregressive (AR) component for past values, the moving average (MA) component for past forecast errors, and the differencing (I) component to account for non-stationary data. It is suitable for time series data with trend and seasonality.
Neural Networks and Machine Learning:
Artificial Neural Networks (ANN): ANNs use interconnected nodes (neurons) to learn and forecast demand patterns from historical data. They can capture complex relationships between variables but require a significant amount of data and computational resources.
Random Forests: Random Forests utilize an ensemble of decision trees to predict demand based on input variables. They handle non-linear relationships, interactions between variables, and provide robust predictions.
Judgmental Forecasting:
Delphi Method: Involves collecting expert opinions and aggregating them iteratively to achieve consensus on demand forecasts. It relies on the knowledge and experience of domain experts.
Market Research and Surveys: Gathering customer feedback, conducting market research studies, and surveys to assess customer preferences and intentions. These insights help in forecasting demand for new products or emerging markets.
Combining Forecasts:
Ensemble Forecasting: Combines forecasts from multiple methods or models to improve forecast accuracy. Techniques like averaging, weighted averaging, or selecting the best forecast based on past performance can be used.
Manufacturers often employ a combination of these forecasting methods based on their specific needs and available data. It's essential to continuously evaluate and refine the forecasting methods used to ensure their effectiveness and accuracy.
B. Scenario Planning and Risk Assessment
Scenario planning and risk assessment are essential components of effective demand forecasting in manufacturing. They help manufacturers anticipate and prepare for potential uncertainties, mitigate risks, and make informed decisions. Here is a detailed explanation of scenario planning and risk assessment:
Scenario Planning:
Scenario planning involves creating and analyzing multiple plausible scenarios that could impact demand. It helps manufacturers envision different future situations and understand their potential consequences. Here's how scenario planning works:
Identify Key Uncertainties: Identify the key uncertainties that could significantly impact demand. These uncertainties can be internal (e.g., production capacity) or external (e.g., economic conditions, market trends, competitor actions).
Develop Scenarios: Create a range of plausible scenarios based on the identified uncertainties. Each scenario represents a different combination of factors, presenting distinct demand patterns. Scenarios can be based on optimistic, pessimistic, or moderate assumptions.
Quantify Scenarios: Assign specific values or ranges to relevant variables within each scenario. For example, consider variations in market demand, input costs, exchange rates, or regulations. Quantify the impact of each variable on demand to assess different demand outcomes.
Forecast Demand for Scenarios: Utilize appropriate forecasting methods to estimate demand under each scenario. Apply forecasting models, historical data, and expert judgment to project demand patterns for different scenarios.
Analyze and Evaluate Scenarios: Assess the implications of each scenario on various aspects, such as production capacity, resource allocation, inventory management, and financial performance. Analyze the sensitivity of key performance indicators to changes in demand under different scenarios.
Develop Action Plans: Develop action plans for each scenario, outlining the necessary adjustments, resource allocation, and contingency measures. Determine strategies for scaling up or scaling down production, managing inventory levels, or adapting marketing and sales approaches.
Monitor and Update Scenarios: Regularly review and update scenarios as new information becomes available. Monitor market conditions, economic indicators, and other relevant factors to assess the likelihood of different scenarios. Adjust forecasts and action plans accordingly.
Risk Assessment:
Risk assessment involves identifying, analyzing, and managing risks associated with demand forecasting. It helps manufacturers identify potential risks that could impact demand accuracy and develop strategies to mitigate them. Here's how risk assessment works:
Identify Risks: Identify potential risks that could affect demand forecasting accuracy. These risks can include demand volatility, supply chain disruptions, raw material price fluctuations, regulatory changes, or competitor actions.
Assess Probability and Impact: Evaluate the probability of each risk occurring and the potential impact on demand forecasts. Categorize risks based on their likelihood and severity. Consider historical data, expert opinions, and industry trends to assess risks.
Prioritize Risks: Prioritize risks based on their significance and potential impact on the business. Focus on risks that have higher probability and greater potential consequences. This helps allocate resources and attention effectively.
Develop Mitigation Strategies: Develop strategies to mitigate identified risks. This can include implementing robust inventory management practices, diversifying suppliers, developing alternative sourcing options, or implementing safety stock measures. Plan contingency measures to respond swiftly if risks materialize.
Implement Monitoring Mechanisms: Establish monitoring mechanisms to track and assess identified risks. Regularly review and update risk assessments based on changing market conditions or new information. Monitor key indicators and triggers that may signal the occurrence or escalation of risks.
Continuously Improve Risk Management: Continuously evaluate the effectiveness of risk management strategies and make necessary adjustments. Learn from past experiences and incorporate lessons learned into future risk assessments. Foster a culture of risk awareness and proactive risk management within the organization.
By incorporating scenario planning and risk assessment into demand forecasting processes, manufacturers can better anticipate uncertainties, make informed decisions, and effectively manage risks. This enables them to adapt to changing market conditions, optimize resource allocation, and improve overall business performance.
C. Agile Production and Supply Chain Management
Agile production and supply chain management are approaches that emphasize flexibility, responsiveness, and adaptability in the manufacturing industry. They enable manufacturers to quickly respond to changing customer demands, market conditions, and unforeseen disruptions. Here is a detailed explanation of agile production and supply chain management:
Agile Production:
Agile production refers to the ability of manufacturing operations to rapidly adjust production processes, capacities, and product offerings to meet dynamic market demands. The focus is on achieving quick turnaround times, reducing lead times, and enabling customization. Here are key elements of agile production:
- Modular and Flexible Manufacturing Systems: Implement modular production systems that allow for easy reconfiguration and scalability. Flexible manufacturing cells, modular assembly lines, and versatile equipment enable quick changes to production processes and layouts to accommodate varying product requirements.
- Cross-Trained and Empowered Workforce: Develop a skilled and cross-trained workforce capable of performing multiple tasks and adapting to changing production needs. Empower employees to make decisions at the operational level, fostering a culture of continuous improvement and innovation.
- Rapid Prototyping and Iterative Design: Utilize rapid prototyping techniques such as 3D printing to quickly produce and test product prototypes. This enables iterative design and facilitates faster product development cycles, reducing time-to-market.
- Collaborative Supplier Relationships: Cultivate strong relationships with suppliers based on collaboration, trust, and information sharing. Establish partnerships that enable quick response to changes in demand, supplier disruptions, or component availability issues.
- Lean Manufacturing Principles: Apply lean manufacturing principles to minimize waste, improve efficiency, and optimize resource utilization. Eliminate non-value-added activities, implement just-in-time (JIT) inventory systems, and focus on continuous flow and pull-based production.
Supply Chain Management:
Agile supply chain management involves the integration and coordination of various supply chain activities to respond rapidly to market changes, customer requirements, and disruptions. The goal is to enhance responsiveness, reduce lead times, and optimize inventory levels. Here are key elements of agile supply chain management:
- Demand-Driven Planning and Forecasting: Utilize demand-driven planning and forecasting techniques to accurately anticipate customer demands. Incorporate real-time data, market insights, and collaborative forecasting with key stakeholders to align supply with demand.
- Flexible and Collaborative Supplier Network: Develop a flexible supplier network that can quickly adapt to changes in demand and supply conditions. Foster collaborative relationships with suppliers, enabling effective communication, shared information, and joint decision-making.
- Agile Inventory Management: Implement agile inventory management practices to reduce excess inventory and optimize stock levels. Utilize techniques such as vendor-managed inventory (VMI), consignment inventory, and real-time inventory tracking to enable better inventory visibility and responsiveness.
- Responsive Production Scheduling: Implement dynamic production scheduling techniques that allow for quick adjustments based on changes in demand, customer priorities, and supply availability. Utilize advanced planning and scheduling (APS) systems to optimize production sequences and minimize changeover times.
- Risk Management and Resilience: Proactively identify and mitigate risks to ensure supply chain resilience. Develop contingency plans, diversify suppliers, and implement risk mitigation strategies to handle disruptions such as natural disasters, geopolitical events, or supply chain bottlenecks.
- Information Technology and Data Analytics: Leverage technology solutions such as advanced analytics, cloud-based systems, and real-time data integration to enable better visibility, information sharing, and decision-making across the supply chain. Utilize data analytics to identify patterns, detect anomalies, and make data-driven decisions.
- Continuous Improvement and Collaboration: Foster a culture of continuous improvement and collaboration within the supply chain. Encourage feedback, conduct regular performance evaluations, and actively seek opportunities for optimization and innovation.
By implementing agile production and supply chain management practices, manufacturers can enhance their ability to quickly respond to changing customer demands, improve operational efficiency, reduce lead times, and effectively manage disruptions. This enables them to stay competitive in dynamic market environments and deliver superior customer satisfaction.
Frequently Asked Questions (FAQs) Associated with Demand Forecasting in Manufacturing
Following, we’ve discussed frequently asked questions (FAQs) associated with demand forecasting in manufacturing. Let’s learn
Que 1: What are the different forecasting methods used in manufacturing?
Various forecasting methods can be used, including:
- Time series analysis techniques (moving averages, exponential smoothing).
- Regression analysis (simple linear regression, multiple regression).
- Seasonal forecasting methods (seasonal decomposition, seasonal exponential smoothing).
- Advanced techniques like Box-Jenkins ARIMA models, neural networks, and machine learning algorithms.
- Judgmental forecasting methods, such as Delphi method or market research surveys.
Que 2: How can manufacturers incorporate external factors in demand forecasting?
Ans: Manufacturers can incorporate external factors by
- Monitoring market trends, economic indicators, and competitor activities.
- Conducting market research and analyzing customer behavior.
- Integrating external data sources, such as industry reports or market intelligence.
- Using qualitative judgment and expert opinions to assess the impact of external factors.
- Applying statistical techniques to quantify the relationship between external factors and demand.
Que 3: How does continuous improvement and evaluation contribute to effective demand forecasting?
Ans: Continuous improvement and evaluation play a vital role in demand forecasting by:
- Assessing forecast accuracy and identifying areas for improvement.
- Analyzing forecast errors and adjusting forecasting methodologies accordingly.
- Incorporating feedback from stakeholders and learning from past forecasting performance.
- Refining forecasting models, processes, and data collection techniques over time.
- Ensuring forecasting practices remain agile and responsive to changing market conditions.
Que 4: What is the role of collaboration between departments in demand forecasting?
Ans: Collaboration between departments, such as sales, marketing, operations, and finance, is crucial for effective demand forecasting. It enables the exchange of information, alignment of goals and objectives, and integration of different perspectives. Collaborative efforts help ensure that demand forecasts are based on comprehensive inputs, market insights, and cross-functional knowledge, leading to more accurate and reliable forecasts.
Que 5: How does incorporating risk assessment and scenario planning improve demand forecasting?
Ans: Incorporating risk assessment and scenario planning enhances demand forecasting by:
- Identifying potential risks and uncertainties that can impact demand.
- Assessing the probability and potential impact of those risks.
- Developing contingency plans and mitigation strategies.
- Considering multiple future scenarios to anticipate different demand outcomes.
- Evaluating the sensitivity of forecasts to changes in market conditions or key variables.
- Enhancing preparedness and enabling proactive decision-making in the face of uncertainties.
Que 6: What are the benefits of using multiple forecasting methods?
Ans: Using multiple forecasting methods offers several benefits, including:
- Diversification of forecasting approaches to capture different aspects of demand.
- Comparison and validation of forecasts from different methods to improve accuracy.
- Identification of patterns, trends, and anomalies that may be missed by a single method.
- Flexibility to select the most appropriate method for different product categories or market conditions.
- Reduction of forecast bias and increased confidence in forecast accuracy.
Que 7: How can manufacturers ensure continuous improvement in demand forecasting?
Ans: Manufacturers can promote continuous improvement in demand forecasting through the following actions:
- Regularly reviewing and evaluating forecast accuracy against actual demand.
- Analyzing forecast errors and identifying the root causes.
- Seeking feedback from stakeholders and incorporating their input for process enhancement.
- Incorporating new data sources and refining forecasting models based on insights gained.
- Investing in training and development of forecasting teams.
- Embracing technological advancements and leveraging advanced analytics for improved forecasting performance.
Que 8: How can demand forecasting support supply chain management?
Ans: Demand forecasting plays a vital role in supply chain management by providing insights into expected customer demand. It helps:
- Optimize procurement, production, and inventory management activities based on forecasted demand.
- Reduce stockouts, excess inventory, and carrying costs.
- Enhance supply chain visibility and coordination with suppliers and partners.
- Support accurate sales and operations planning.
- Facilitate effective capacity planning and resource allocation.
- Improve customer satisfaction through timely and reliable product availability.
Wrapping Up
In conclusion, demand forecasting in manufacturing presents various challenges that can impact operational efficiency and profitability.
However, implementing best practices and strategies can help mitigate these challenges. Key takeaways include the importance of data accuracy, incorporating external factors, leveraging advanced analytics and machine learning techniques, and fostering collaboration between departments.
By adopting these practices, manufacturers can enhance their forecasting capabilities, optimize inventory management, reduce costs, and meet customer demand more effectively, ultimately driving success in a competitive market.
How Deskera Can Assist You?
Deskera ERP and MRP systems help you to keep your business units organized. The system's key features of demand forecasting with Deskera include as follows:
- Establish Demand Forecasting Process
- Automate Data Collection
- Monitor Market Trends
- Analyze Historical Data
- Estimate Future Demand
- Adjust Production Levels
- Manage Supply Chain...and much more
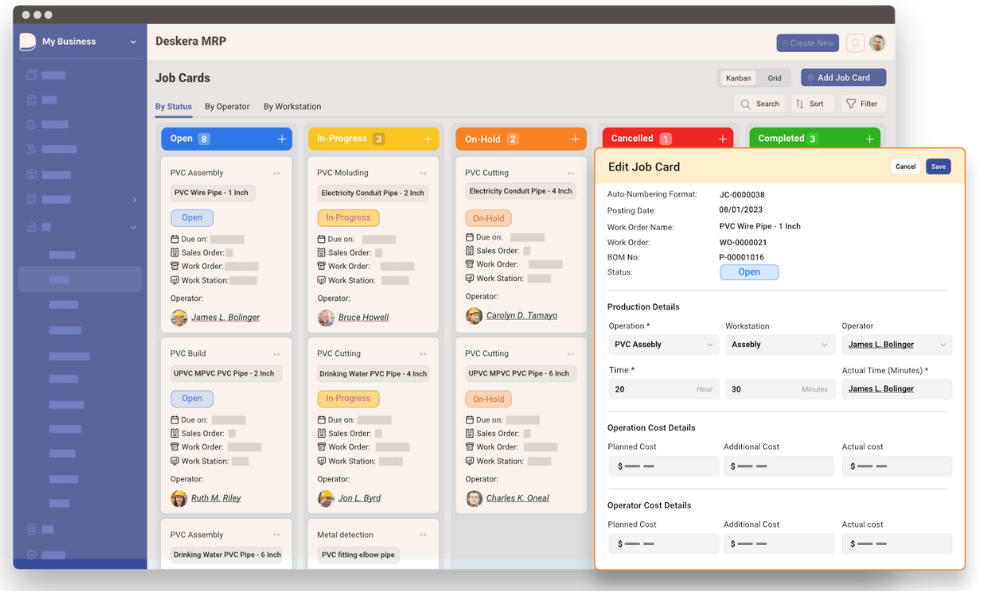
- Deskera's integrated financial planning tools enable investors to better plan and track their investments. It can assist investors in making faster and more accurate decisions.
- Deskera Books allows you to better manage your accounts and finances. Maintain good accounting practices by automating tasks like billing, invoicing, and payment processing.
- Deskera CRM is a powerful solution that manages your sales and helps you close deals quickly. It not only enables you to perform critical tasks like lead generation via email, but it also gives you a comprehensive view of your sales funnel.
- Deskera People is a straightforward tool for centralizing your human resource management functions.
Final Takeaways
We've arrived at the last section of this guide. Let's have a look at some of the most important points to remember:
- Demand forecasting in manufacturing refers to the process of estimating future customer demand for a manufacturer's products or services. Furthermore, it involves analyzing historical data, market trends, and other relevant factors to predict the quantity of products that will be needed within a specific time frame.
- Demand forecasting serves as a foundation for strategic decision-making in manufacturing. It enables manufacturers to identify market trends, anticipate changes in customer preferences, and make informed decisions about product development, expansion, market entry, or discontinuation.
- Several external factors can influence demand but are beyond the manufacturer's control. Examples include regulatory changes, geopolitical events, natural disasters, economic recessions, or shifts in global trade policies. These factors can significantly impact customer demand, supply chain dynamics, and market conditions, making it challenging to predict and incorporate their effects into demand forecasts.
- Identify and gather relevant data sources that provide insights into customer demand. This includes historical sales data, customer orders, market research, website analytics, social media data, and industry reports. Incorporate internal and external data sources to capture a comprehensive view of demand drivers.
- Utilize appropriate forecasting models based on the characteristics of the data and the specific needs of the manufacturing industry. Common forecasting models include moving averages, exponential smoothing, regression models, and machine learning algorithms. Choose models that align with the nature of the data and have a proven track record of accuracy.
- Develop action plans for each scenario, outlining the necessary adjustments, resource allocation, and contingency measures. Determine strategies for scaling up or scaling down production, managing inventory levels, or adapting marketing and sales approaches.
- Apply lean manufacturing principles to minimize waste, improve efficiency, and optimize resource utilization. Eliminate non-value-added activities, implement just-in-time (JIT) inventory systems, and focus on continuous flow and pull-based production.
- Proactively identify and mitigate risks to ensure supply chain resilience. Develop contingency plans, diversify suppliers, and implement risk mitigation strategies to handle disruptions such as natural disasters, geopolitical events, or supply chain bottlenecks.
Related Articles
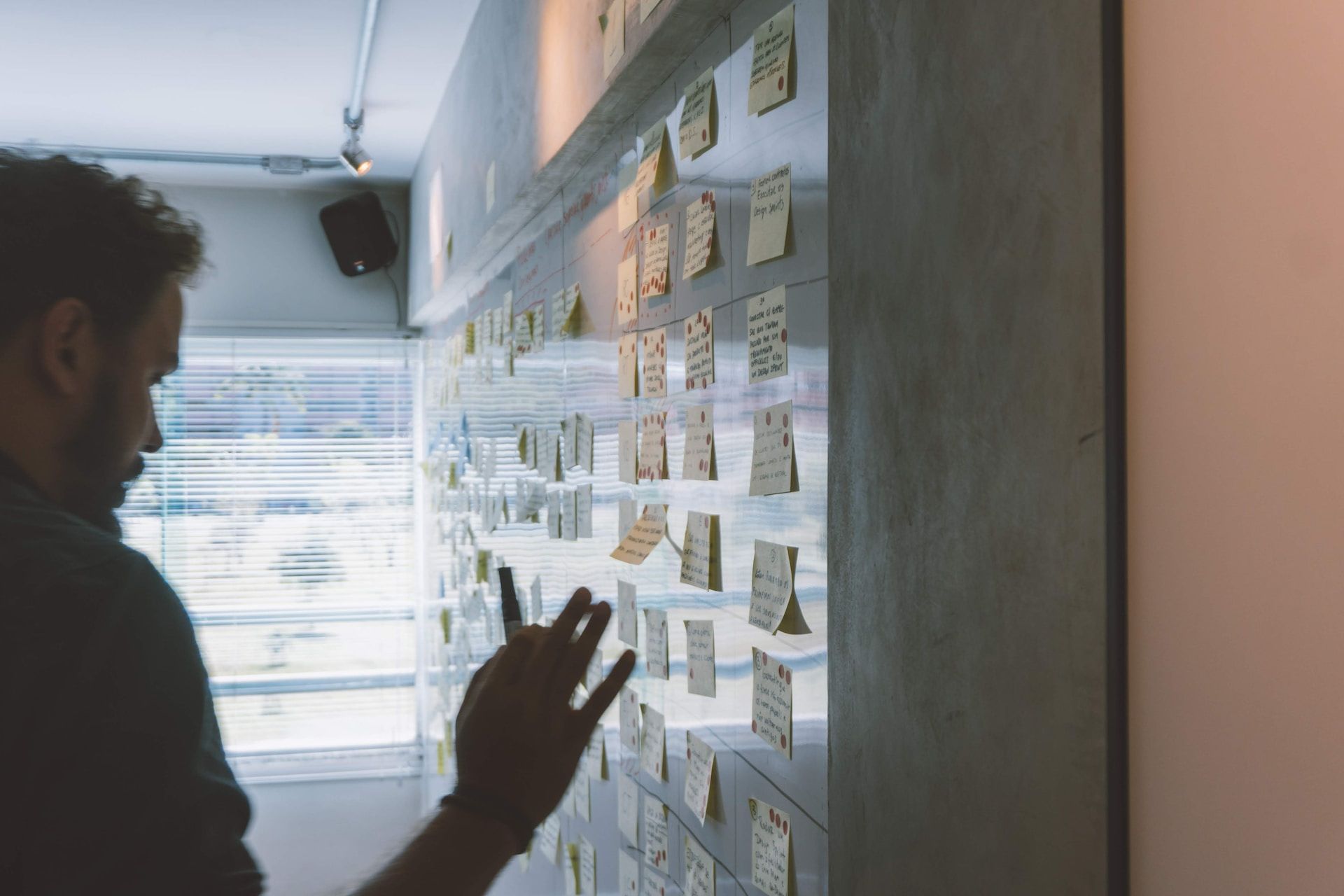
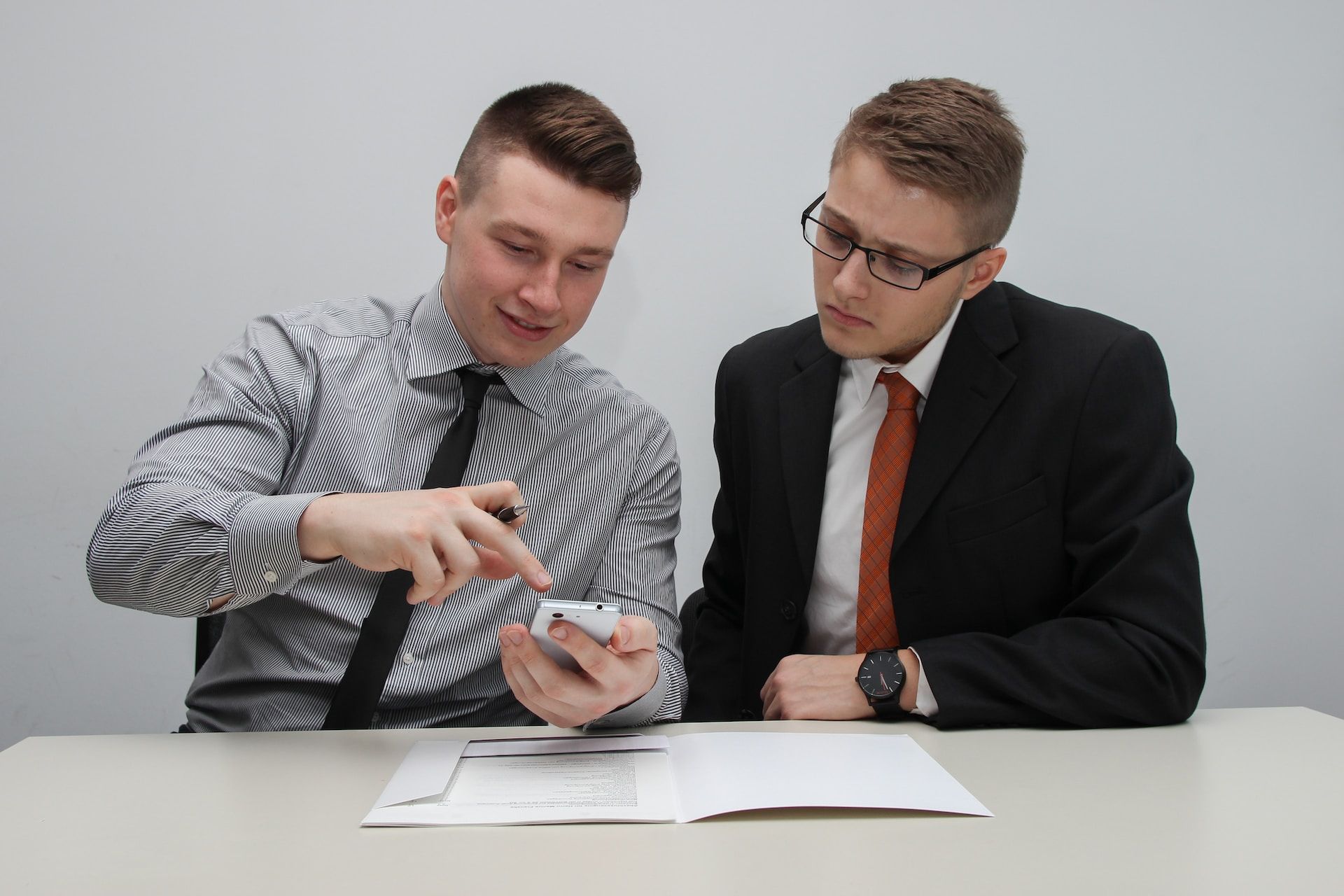
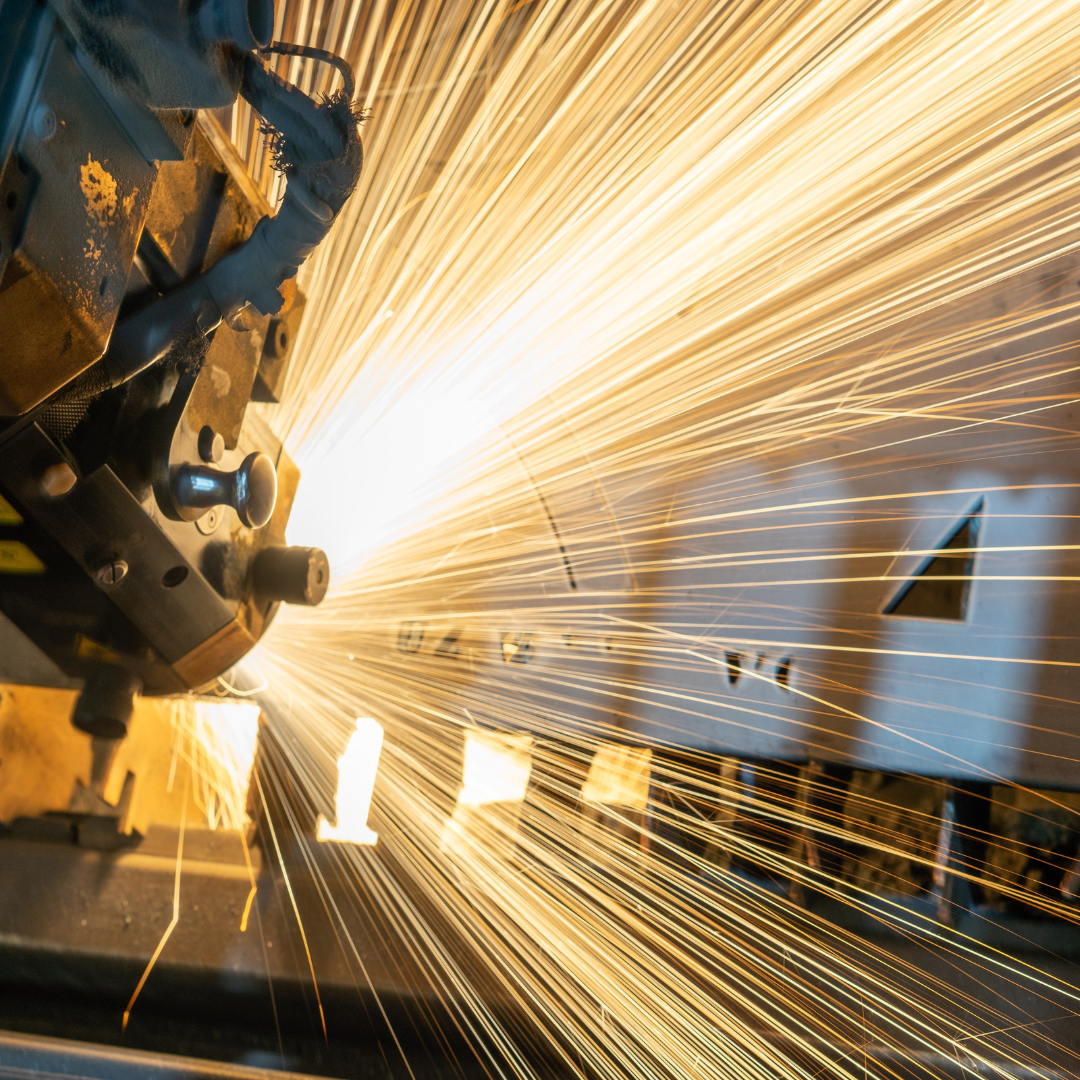
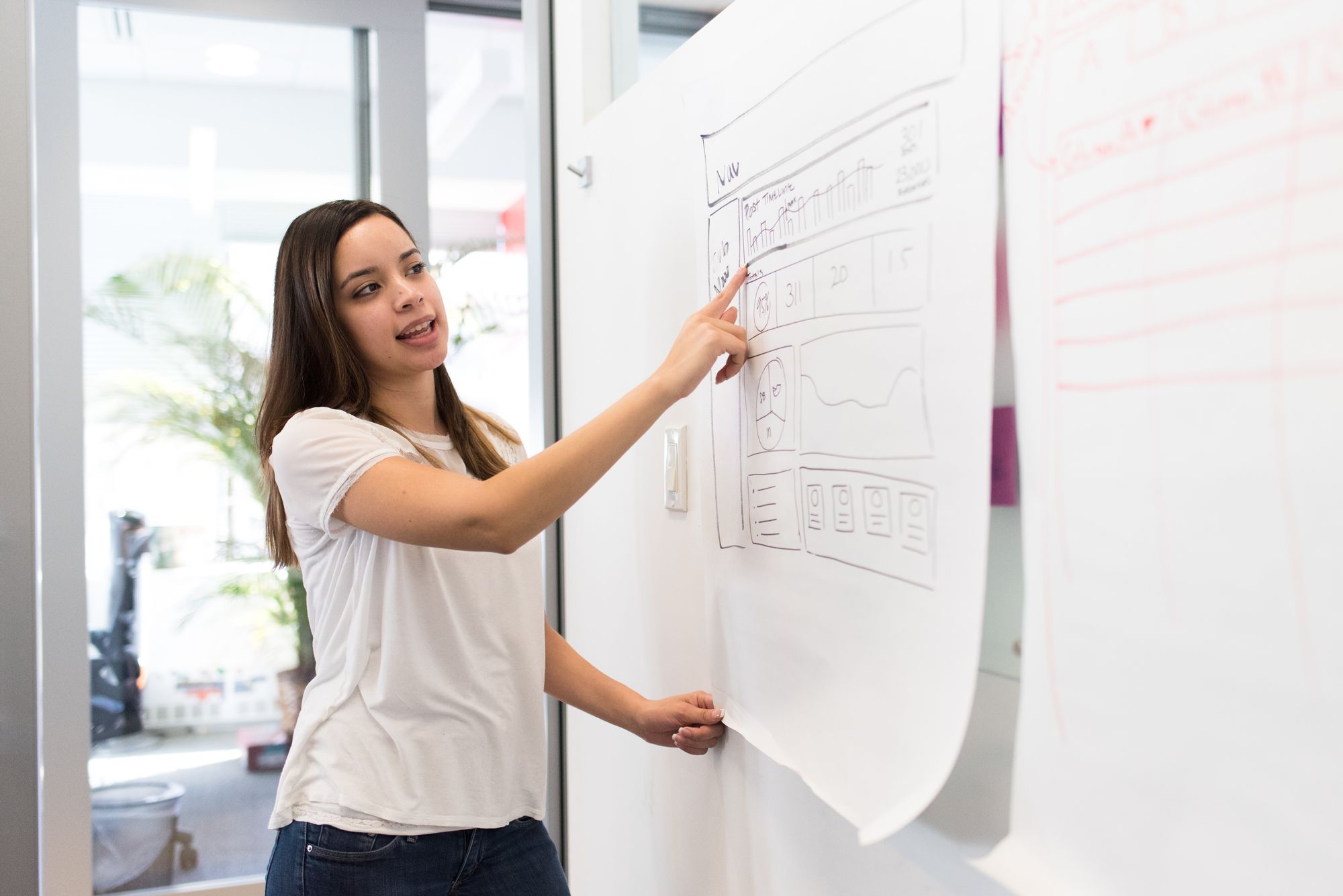
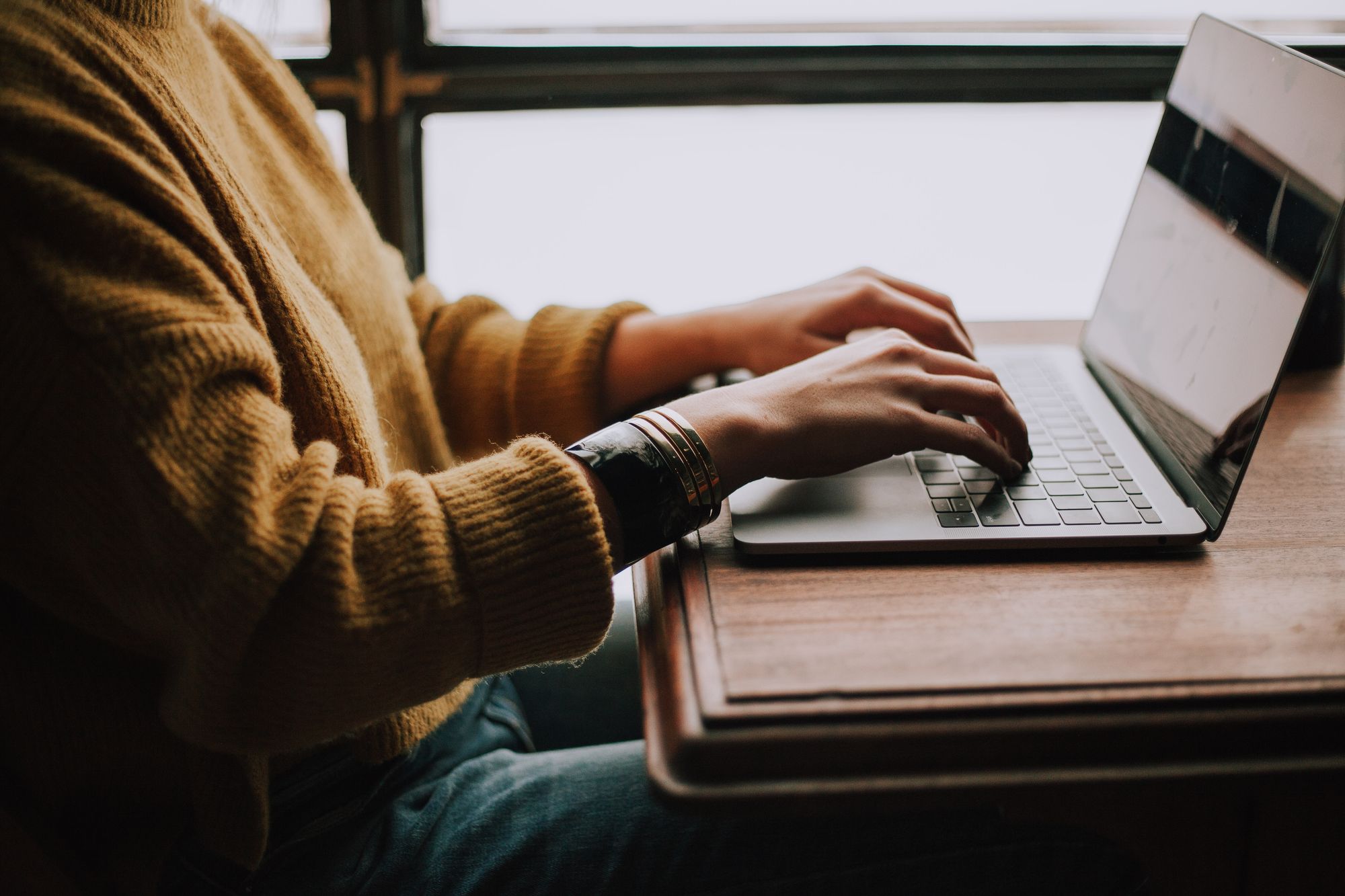